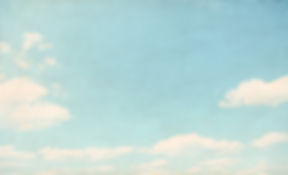
Welcome
Research Interests
-
Time Series and Spectral Analysis
-
Computational Statistics and Bayesian Inference
-
I am interested in all aspects of statistical methods in neuroscience. Recently, my work has focus on analysis of EEG data, and pre-processing fMRI, but I have begun learning neural spike train data, i.e. Gaussian process model for firing rate. I also wish to learn about other statistical issuses beside neuroscience.
Projects and Publications
1) A Multivariate Statistival Inference for the Analysis of Neuronal Spiking Rates.
In this work, we propose a series of comparative statistical inferences that may be used to distinguish the firing patterns of a population of neurons recorded under two experimental conditions and classify neurons based on their differential intensity rates. To address these questions, we utilized features of multivariate Gaussian distributions and hierarchical clustering techniques. This in turn, would allow us to simulate a large number of firing intensity curves from the underlying multivariate distributions for further inferential steps. Applying simulation-based methods in this work, we were able to construct a 95% confidence interval for the differences between two curves fitted to each neuron. We found that a considerable portion of 139 studied neurons demonstrated significant differences throughout the entire experimental time window. Using two different metrics of distance between the simulated curves, a Kullback-Leibler divergence, and a bin-wise method, we constructed a 95% confidence interval for the mean of six clusters of the 139 difference curves.